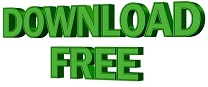
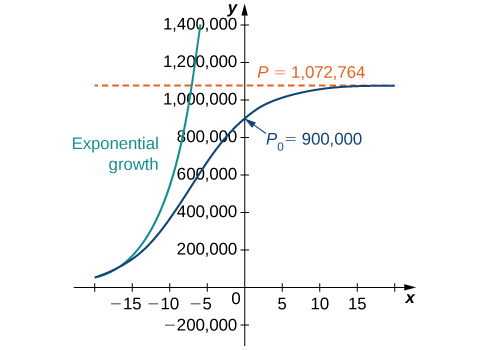
You can find more Excel tutorials on this page. For example if x = 4 then we would predict that y = 23.34: We can also use this equation of the curve to predict the value of the response variable based on the predictor variable. output mode, see the explanation of the Input/Output mode setting under Using the. This R-squared is considerably higher than that of the previous curve, which indicates that it fits the dataset much more closely. Metric Conversion Calculations (all models except fx-9860GII s). The R-squared for this particular curve is 0.9707. We can also increase the order of the Polynomial that we use to see if a more flexible curve does a better job of fitting the dataset.įor example, we could choose to set the Polynomial Order to be 4: The R-squared for this particular curve is 0.5874. It’s better to just do the logistic regression. It is still recommended sometimes, but it’s an ad-hoc way of fitting a binary outcome into a normal model. The R-squared tells us the percentage of the variation in the response variable that can be explained by the predictor variables. Square root of arcsin is an alternative to logistic regression, but it’s arcane. This produces the following curve on the scatterplot: In the above equation, there is log(1+something) which is always positive and we want a value of W which minimizes the complete equation(for us this value. Then check the boxes next to Display Equation on chart and Display R-squared value on chart. optimization problem logistic regression. In the window that appears to the right, click the button next to Polynomial. In the dropdown menu, click the arrow next to Trendline and then click More Options: Then click the + sign in the top right corner. Next, click the Insert tab along the top ribbon, and then click the first plot option under Scatter: Next, let’s create a scatterplot to visualize the dataset.įirst, highlight cells A2:B16 as follows: Step 1: Create the Dataįirst, let’s create a fake dataset to work with: This tutorial provides a step-by-step example of how to fit an equation to a curve in Excel. The research findings of this paper help traffic management departments develop and optimize traffic control schemes, which can be applied to Intelligent Vehicle Infrastructure Cooperative Systems (IVICS) dynamic warning.Often you may want to find the equation that best fits some curve for a dataset in Excel.įortunately this is fairly easy to do using the Trendline function in Excel. After normalizing and dimensionality reduction of the vehicle trajectory dataset, Naive Bayes, Logistic Regression, and Gradient Boosting Decision Tree (GBDT) models were selected for traffic conflict prediction, and the experiments showed that the GBDT model outperforms two remaining models in terms of prediction accuracy, precision, false-positive rate (FPR) and Area Under Curve (AUC). In this case one’s assumptions about the growth of the population include a maximum size beyond which the population cannot expand. Specifically, TTC was used to capture the collision risk severity, and ML extracted vehicle trajectory features. The Logistic Differential Equation A more realistic model for population growth in most circumstances, than the exponential model, is provided by the Logistic Differential Equation.

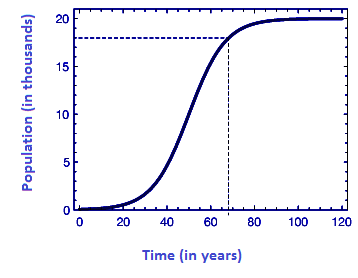
The model can accurately learn various features, such as vehicle operation characteristics, risk and conflict distributions, and physical zoning characteristics in the weaving area. In this paper, a potential collision risk identification and conflict prediction model based on extending Time-to-Collision-Machine Learning (TTC-ML) for multi-lane weaving zone was proposed. However, most of the existing studies focus only on highways, not on multi-lane weaving areas. The logistic classification model has the following characteristics: the output variable can be equal to either 0 or 1 the predicted output is a number between 0 and 1 as in linear regression, we use a vector of estimated coefficients to compute, a linear combination of the input variables unlike in linear regression, we transform using a. Crash risk identification and prediction are expected to play an important role in traffic accident prevention.
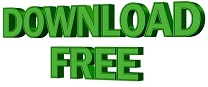